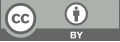
ChatGPT v.s. media bias: A comparative study of GPT-3.5 and fine-tuned language models
- 1 Shenzhen College of International Education
- 2 Duke University
* Author to whom correspondence should be addressed.
Abstract
In our rapidly evolving digital sphere, the ability to discern media bias becomes crucial as it can shape public sentiment and influence pivotal decisions. The advent of large language models (LLMs), such as ChatGPT, noted for their broad utility in various natural language processing (NLP) tasks, invites exploration of their efficacy in media bias detection. Can ChatGPT detect media bias? This study seeks to answer this question by leveraging the Media Bias Identification Benchmark (MBIB) to assess ChatGPT's competency in distinguishing six categories of media bias, juxtaposed against fine-tuned models such as Bidirectional and Auto-Regressive Transformers (BART), Convolutional Bidirectional Encoder Representations from Transformers (ConvBERT), and Generative Pre-trained Transformer 2 (GPT-2). The findings present a dichotomy: ChatGPT performs at par with fine-tuned models in detecting hate speech and text-level context bias, yet faces difficulties with subtler elements of other bias detections, namely, fake news, racial, gender, and cognitive biases.
Keywords
media bias detection, large language model, comparative analysis
[1]. Wessel, M., Horych, T., Ruas, T., Aizawa, A., Gipp, B., & Spinde, T. Introducing MBIB--the first Media Bias Identification Benchmark Task and Dataset Collection. 2023, arXiv preprint arXiv:2304.13148.
[2]. Puglisi, R., & Snyder Jr, J. M. Empirical studies of media bias. 2015, Handbook media Econ. 1, 647-667.
[3]. Hamborg, F., Donnay, K., & Gipp, B. Automated identification of media bias in news articles: an interdisciplinary literature review. 2019, Inter. J. Digital Libraries, 20(4), 391-415.
[4]. Groseclose, T., & Milyo, J. A measure of media bias. 2015, The Quarterly J. Economics, 120(4), 1191-1237.
[5]. Papacharissi, Z., & de Fatima Oliveira, M. News frames terrorism: A comparative analysis of frames employed in terrorism coverage in US and UK newspapers. 2008 The Inter. J. press, 13(1), 52-74.
[6]. Smith, J., McCarthy, J. D., McPhail, C., & Augustyn, B. From protest to agenda building: Description bias in media coverage of protest events in Washington, DC. 2001, Social Forces, 79(4), 1397-1423.
[7]. Van der Pas, D. J., & Aaldering, L. Gender differences in political media coverage: A meta-analysis.2020 J. Communication, 70(1), 114-143.
[8]. D’Alonzo, S., & Tegmark, M. Machine-learning media bias. 2022 Plos one, 17(8), e0271947.
[9]. Spinde, T., Krieger, J. D., Ruas, T., Mitrović, J., Götz-Hahn, F., Aizawa, A., & Gipp, B. Exploiting transformer-based multitask learning for the detection of media bias in news articles. 2022, Inter. Conf. Virtual Event, 225-235.
[10]. Gangula, R. R. R., Duggenpudi, S. R., & Mamidi, R. Detecting political bias in news articles using headline attention. 2019 Anal. Interpret. Neur. Net. NLP 77-84.
[11]. Thota, A., Tilak, P., Ahluwalia, S., & Lohia, N. Fake news detection: a deep learning approach. 2018, SMU Data Science Review, 1(3), 10.
[12]. Jiao, W., Wang, W. X., Huang, J. T., Wang, X., & Tu, Z. P. Is ChatGPT a good translator? Yes with GPT-4 as the engine. 2023 arXiv preprint arXiv:2301.08745.
[13]. Qin, C., Zhang, A., Zhang, Z., Chen, J., Yasunaga, M., & Yang, D. Is ChatGPT a general-purpose natural language processing task solver?.2023 arXiv preprint arXiv:2302.06476.
[14]. Yang, X., Li, Y., Zhang, X., Chen, H., & Cheng, W. Exploring the limits of chatgpt for query or aspect-based text summarization. 2023 arXiv preprint arXiv:2302.08081.
[15]. Wang, Z., Xie, Q., Ding, Z., Feng, Y., & Xia, R. Is ChatGPT a good sentiment analyzer? A preliminary study. 2023 arXiv preprint arXiv:2304.04339.
[16]. Lewis, M., Liu, Y., Goyal, N., Ghazvininejad, M., Mohamed, A., Levy, O., Zettlemoyer, L. Bart: Denoising sequence-to-sequence pre-training for natural language generation, translation, and comprehension. 2019, arXiv preprint arXiv:1910.13461.
[17]. Jiang, Z. H., Yu, W., Zhou, D., Chen, Y., Feng, J., & Yan, S. Convbert: Improving bert with span-based dynamic convolution. 2023 Adv. Neur. Infor. Proc. Sys. 33, 12837-12848.
[18]. Radford, A., Wu, J., Child, R., Luan, D., Amodei, D., & Sutskever, I. Language models are unsupervised multitask learners. 2019, OpenAI blog, 1(8), 9.
Cite this article
Wen,Z.;Younes,R. (2023).ChatGPT v.s. media bias: A comparative study of GPT-3.5 and fine-tuned language models.Applied and Computational Engineering,21,249-257.
Data availability
The datasets used and/or analyzed during the current study will be available from the authors upon reasonable request.
Disclaimer/Publisher's Note
The statements, opinions and data contained in all publications are solely those of the individual author(s) and contributor(s) and not of EWA Publishing and/or the editor(s). EWA Publishing and/or the editor(s) disclaim responsibility for any injury to people or property resulting from any ideas, methods, instructions or products referred to in the content
About volume
Volume title: Proceedings of the 5th International Conference on Computing and Data Science
© 2024 by the author(s). Licensee EWA Publishing, Oxford, UK. This article is an open access article distributed under the terms and
conditions of the Creative Commons Attribution (CC BY) license. Authors who
publish this series agree to the following terms:
1. Authors retain copyright and grant the series right of first publication with the work simultaneously licensed under a Creative Commons
Attribution License that allows others to share the work with an acknowledgment of the work's authorship and initial publication in this
series.
2. Authors are able to enter into separate, additional contractual arrangements for the non-exclusive distribution of the series's published
version of the work (e.g., post it to an institutional repository or publish it in a book), with an acknowledgment of its initial
publication in this series.
3. Authors are permitted and encouraged to post their work online (e.g., in institutional repositories or on their website) prior to and
during the submission process, as it can lead to productive exchanges, as well as earlier and greater citation of published work (See
Open access policy for details).