Abstract
On 4–6 December 2015, storm Desmond caused very heavy rainfall in Northern England and Southern Scotland which led to widespread flooding. A week after the event we provided an initial assessment of the influence of anthropogenic climate change on the likelihood of one-day precipitation events averaged over an area encompassing Northern England and Southern Scotland using data and methods available immediately after the event occurred. The analysis was based on three independent methods of extreme event attribution: historical observed trends, coupled climate model simulations and a large ensemble of regional model simulations. All three methods agreed that the effect of climate change was positive, making precipitation events like this about 40% more likely, with a provisional 2.5%–97.5% confidence interval of 5%–80%. Here we revisit the assessment using more station data, an additional monthly event definition, a second global climate model and regional model simulations of winter 2015/16. The overall result of the analysis is similar to the real-time analysis with a best estimate of a 59% increase in event frequency, but a larger confidence interval that does include no change. It is important to highlight that the observational data in the additional monthly analysis does not only represent the rainfall associated with storm Desmond but also that of storms Eve and Frank occurring towards the end of the month.
Export citation and abstract BibTeX RIS

Original content from this work may be used under the terms of the Creative Commons Attribution 3.0 licence.
Any further distribution of this work must maintain attribution to the author(s) and the title of the work, journal citation and DOI.
1. Introduction
Atlantic storm Desmond passed over Ireland, Scotland and northern England from early Friday 4 December to early Sunday 6 December 2015, causing very heavy rainfall and gale-force winds. Its severity is illustrated by reports stating that the UK provisionally set a new record for the greatest 24 h rainfall recorded with 341.1 mm in Honister, Cumbria between 18:30, 4 December and 18:30, 5 December (all times are in UTC, which is also local time). The UK Met Office issued a rare Red 'take action' warning (www.metoffice.gov.uk/news/releases/archive/2015/storm-desmond-red-warning)—the first since 12 February 2014—for parts of Cumbria and the Scottish Borders during the passage of this storm. The heavy rainfall indeed led to widespread flooding in these regions. It was reported that about 5000 homes and businesses were flooded and 60000 people experienced power cuts (Daily Telegraph 2015, CNN 2015). In the same month the UK Met Office issued a Red warning again on 26 December 2015 for storm Eve (www.metoffice.gov.uk/news/releases/2015/red-warning) with Amber and Yellow warnings in the same parts of the country affected by the rainfall following storm Desmond. The month of December 2015 closed with a third storm, Frank, bringing in again strong winds and extreme rainfall over Northwest Scotland. The extreme rainfall events have led many to question whether climate change played a role, especially since there have been several large floods over the last decades. These questions were asked on the days following the individual event, when usually no scientific information specific to the event is available. In similar cases, answers are usually given based on opinions or generalities rather than a scientific analysis of the event. At the time of storm Desmond we provided a real-time analysis discussion paper (van Oldenborgh et al 2015), indicating that anthropogenic climate change did play a small but significant role. Now that we have more data available we revisit the analysis and show that the first estimate of the overall role anthropogenic climate change played in the likelihood of this type of heavy precipitation events to occur lies well within the confidence interval of the updated results, highlighting that it is possible for these kinds of event to provide robust estimates on a time scale of a week.
Although the Clausius–Clapeyron relation points to the possibility of about 6% K−1 more water vapour in a warmer atmosphere, this is not the only factor influencing heavy precipitation as changes in the atmospheric circulation may also play an important role (Otto et al 2016). Hence, results addressing the question of attribution depend on the region and season. Earlier work found no trend in the chance of heavy precipitation related to the Thailand floods (van Oldenborgh et al 2012) or the Elbe and Danube floods (Schaller et al 2014), but a strong increase in Southern France (Vautard et al 2015) and the US central Gulf Coast (van der Wiel et al 2017).
Over Western Europe, both the observations and the models show a shift to more frequent westerly circulation types in winter. This has been shown to increase mean winter precipitation in Europe (van Haren et al 2013b), winter precipitation extremes over the Rhine basin (van Haren et al 2013a) and over Southern England (Schaller et al 2016). This shift has been linked to the increase in temperature contrast between the warming hole in the North Atlantic associated with the weakening Atlantic Meridional Overturning Circulation (Drijfhout et al 2012) and the increase in tropospheric temperature that is advected from the upper tropospheric heating in the tropics with the Hadley circulation (Haarsma et al 2013). It is unclear a priori whether this also affects extreme precipitation in Northern England/Southern Scotland, as trends proportional to global mean surface temperature (GMST) in the North Atlantic Oscillation (NAO) are small both in observations (r = −0.05 in October–February) and climate models (Kirtman et al 2013).
We investigated the precipitation leading to the UK floods of early December 2015 using several independent methods of probabilistic event attribution (Allen 2003): a statistical analysis of the observations (van Oldenborgh 2007), trend detection in a coupled climate model (similar to Lewis and Karoly 2013), analyses of the difference between the actual climate and one without anthropogenic emissions in atmosphere-only general circulation model (Christidis et al 2013), as well as analyses using a very large ensemble of a regional climate model (approximately 500 ensemble members per ensemble) designed for event attribution (Massey et al 2015). The observational analysis gives a probability p1 of a similar event occurring in the current climate, typically expressed as a return period τ1 = 1∕p1. This can in principle also be estimated from the models, but depends sensitively on model biases and bias correction, hence we only estimate the return period of the event from the observations and use this estimate as the event definition. All methods provide changes in the return period, p1∕p0 = τ0∕τ1. However, this measure answers subtly different questions in the different methods (Stott et al 2016): how much the probability changed due to the fitted trend for the observations, due to temporal changes in all forcings in the coupled model and due to anthropogenic forcings in the large ensemble. If the trend is completely due to anthropogenic forcings, the changes in return period will be the same for all methods, provided these forcings are well represented in the models. In the UK winter this is, as far as we know, a reasonable approximation. The influence of natural forcings could be impacting the NAO and hence storminess in the UK (Ineson et al 2011), but solar forcings have been found to have very little influence (van Oldenborgh et al 2013a) and there were no climatologically significant volcanic eruptions the last few years. Low-frequency natural variations also play a minor role here: precipitation extremes are not significantly correlated to the Atlantic Multidecadal Oscillation or Pacific Decadal Oscillation at p < 0.1 over 80 years of observations. The largest uncertainties arise from the random weather, which affects all three methods.
We revisit the real-time analysis here to give better estimates of the return period, which was estimated to be 1 in 100 years (van Oldenborgh et al 2015) and impact of climate change on the event. We also look briefly at a second event definition to account for the fact that storm Desmond did not happen in isolation, the average precipitation over three Northern England/Southern Scotland regions that saw record monthly rainfall in December 2015. The main focus of the paper is however on storm Desmond.
Results for the real-time analysis are not listed in the main text in order not to confuse the numbers. However, they are compared with the revised numbers in the Discussion (section 6) and in table 1.
Table 1. Overview of estimates of risk ratios at the point when the real-time analysis was made compared to the results in this study with more station data (and revised return period) and updated observed and model data.
Real-time estimate | Revised estimate | Method |
---|---|---|
0.3−2.1 | 0.53−1.89 | Observed two day rainfall North West England |
1.8–4 | 1.61–42.89 | Observed two day rainfall Southern Scotland |
1.3–2.8 | 1.49–18.53 | Observed two day rainfall for entire region |
1.1–1.8 | 1.13–1.55 | EC-Earth |
- | 0.98–1.51 | HadGEM3-A |
1.05–1.4 | 1.25–3.48 | weather@home |
Figure 1. Precipitation totals (mm) for (a) 5 December 2015, storm Desmond, and (b) December 2015 total from the E-Obs 0.25° dataset.
Download figure:
Standard image High-resolution image2. Event definition
2.1. Storm Desmond
Most rain fell in Northwest England (figure 1(a), with orographically-driven maxima in Cumbria and Southern Scotland. The rain mostly fell from 18:30 on 4 December to 09:00 on 6 December. This implies that the 24 h period starting from midnight on 4 December (00:00–00:00) captured most of the precipitation, but the 09:00–09:00 rain stations only show the full extent by adding the precipitation recorded on 5 and 6 December. The UK Met Office reported two-day sums of more than 170 mm at four stations (http://blog.metoffice.gov.uk/2015/12/06/wind-and-rain-records-for-storm-desmond/). However, stations very close to these stations received much less rain, due to the mountainous terrain. We therefore use daily precipitation for 00:00–24:00 datasets. When 00:00–24:00 data is not available, we use two-daily precipitation to compare the event itself to its historical record.
We investigated the event at two spatial scales to capture both the orography-driven localised events of very high rainfall and, to increase confidence, larger scale events of high rainfall in larger-area model simulations. The main attribution results are given for a large area with heavy precipitation, defined as the land area of 54–57° N, 6° W–2°W. This area encompasses the two regions Northern England and Southern Scotland as defined in the UK Met Office HadUKP observations http://hadobs.metoffice.gov.uk/hadukp/). Results were checked against smaller areas and local station data. Over the large area, the ERA-Interim reanalysis gives an average precipitation of 25.2 mm on 5 December 2015 (00:00–00:00). In the Netherlands, with similar synoptic weather events and more publically available station data, at a scale of 200 km, ERA-Interim precipitation is strongly correlated with observations (r = 0.95) with an underestimation of about 10%. Precipitation averaged over smaller areas such as the basins of the rivers that flooded, and indeed point data at rain gauges, are assumed to have similar changes in the probability of extreme precipitation due to global warming. The extremes themselves do vary with spatial scale, but the ratios of extremes at different scales are assumed to be constant in time. For large-scale winter precipitation events such as storm Desmond we know of no evidence that would contradict this assumption.
2.2. Monthly context
After having observed the two subsequent storms following Desmond, Eve and Frank, we additionally look at the whole of December precipitation. The results shown here represent Northern England/Southern Scotland precipitation for which we used the average of precipitation in three smaller regions, Northwest England, Northeast England and Southern Scotland. The December 2015 total rainfall, averaged over the three regions, was 215 mm in ERA-Interim for the large area (54–57° N, 6–2° W).
3. Methodology
We use the method of the risk-based probabilistic event attribution (Otto 2017) (Stott et al 2016) based on the assessment of possible weather events under current and preindustrial or counterfactual climate conditions to estimate the occurrence frequency of the event under different conditions. In essence, every extreme weather event is unique and always the result of a combination of external drivers, natural and human induced, as well as internal climate variability and noise; it is therefore impossible to say that an event could not have occurred without anthropogenic influence. However, we can identify whether and to what extend the presence of an external driver such as anthropogenic climate change has altered the likelihood of the occurrence of an extreme weather event. We do this by simulating possible weather under current climate conditions to identify the likelihood of occurrence of an event in question in today's climate and compare this with the likelihood of occurrence of the same kind of event in a counterfactual climate with the human-induced drivers removed. Estimating the likelihood of occurrence of an extreme weather event and thus its return time can be undertaken either on the basis of observed or reanalysis data (here we use the HadUKP rainfall series as well as ERA-Interim data and station data from Eskdalemuir in Southern Scotland (55.27°N, 3.18°W) or on the basis of climate model simulations of possible weather in the current climate (here EC-Earth, HadGEM3-A and weather@home). Each method has advantages and disadvantages. Observations are less biased compared to necessarily imperfect model simulations but records are often short and sparse (with only one station, Eskdalemuir, in the area providing data openly) and thus require assumptions about the properties of the underlying distribution to be made to infer the return times of rare events. In particular, atmosphere-only models can be used to simulate large ensembles, thus allowing for the statistics of rare events to be assessed without further assumptions about the statistical properties of the distribution of the event in question. Sippel et al (2015) compared in perfect model experiments both methods and find that the empirical model distributions do not always match with statistical modeling on a shorter subset of the data, in particular when simulating rainfall. Despite these difficulties of comparing methods, the multi-method approach used here to quantify the role of anthropogenic climate change is the only way to assess confidence in the results (Stott et al 2016).
4. Storm Desmond
4.1. Observational analysis
There are two relatively long (daily data available from 1931) area-averaged daily time series available from the UKMet Office in the areas with severe precipitation during storm Desmond in 2015: Northwest England (15 stations) and Southern Scotland (nine stations) precipitation (for data and maps see http://hadobs.metoffice.gov.uk/hadukp/). We analysed these to investigate trends in daily and two-daily sums of precipitation. Since the underlying station data are obtained from different networks operated by various agencies and have to undergo quality control, the area averages are only publicly released the following month. The observations for December 2015 were therefore not available for the real-time analysis (van Oldenborgh et al 2015).
Now, these data are available: 19.4 mm for Northwest England (33.1 mm in two days) and 34.0 mm for Southern Scotland (60.0 mm in two days). The analysis will mostly focus on the two day values in the observations because the time series are 09:00–09:00 and thus the one-day values do not capture the entire event. In the models, data is recorded from 00:00–00:00, so we will use 24 hour precipitation in that case.
In addition to these area averages, there is only one publicly available precipitation series of station data in the area, Eskdalemuir in Southern Scotland, which recorded 75.7 mm on the morning of 5 December 2015 and 139.86 mm over the two days. Again the observational analysis will focus on two-daily values, for similar reasons as mentioned above.
Figure 2. The annual maximum of two-daily average precipitation amount over October–February in (a) Northwest England and (b) Southern Scotland precipitation from the UKMet Office, 1931–2016, plotted against the smoothed global mean surface temperature (GMST) anomaly in K. The thick line is the position parameter μ and the thin lines are the scale parameter σ and 2σ above it (cf equation 1) The green square denotes the event of December 2015. (c) and (d) Gumbel plots of the same values and GEV fit. The red lines indicate the fit in the current climate (T' = 0.74 K), the blue ones in the climate of the beginning of the time series (T' = −0.16K). The stars denotes the observed maxima, shifted up with the fitted trend to 2015 (red) or down to the beginning of the time series (blue). The two day observed value during storm Desmond is indicated by the green horizontal line.
Download figure:
Standard image High-resolution imageFigure 3. As figure 2 but now for two-daily average precipitation at Eskdalemuir, Scotland. Data source: Klein Tank et al (2002).
Download figure:
Standard image High-resolution imageFigure 4. As figure 2 but now for maximum daily precipitation in the EC-Earth experiment 1860–2016. The observed storm Desmond value is taken from ERA-Interim (25.15 mm, shifted to account for model bias).
Download figure:
Standard image High-resolution imageThe two-daily maxima occurring over the period October–February were computed for each year. This encompasses the season of heavy large-scale precipitation in this area and excludes the season of heavy thunderstorms in the (late) summer. Adding the months of October and November increases the signal-to-noise ratio greatly. These maxima were fitted to a generalised extreme value distribution function (GEV), as is appropriate for block maxima (Coles 2001). The GEV depends on time by scaling it with the low-pass filtered global mean temperature anomaly T' (GISTEMP, Hansen et al 2010), a proxy for anthropogenic climate change. The cumulative distribution function
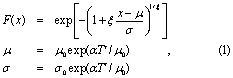
is fitted to the observations using a maximum likelihood method varying α, μ0, σ0 and ξ. Uncertainties are estimated with a non-parametric bootstrap. The results are shown in figure 2 for the two area averages and in figure 3 for Eskdalemuir. The horizontal line denotes the observed precipitation for storm Desmond in the areas.
The Northwest England region shows no trend in the maximum daily precipitation over October–February, with a 95% uncertainty margin on the change in return periods of these extremes between beginning and end of the time series of a factor 0.53–1.89 (1 indicates no change). In Southern Scotland there is a strong positive trend in precipitation, giving an increase in probability of a factor of 1.62–42.89 (95% confidence interval, best estimate 5.14) that what it used to be at the beginning of the series, 1931. This is due to large extent to a heavy precipitation event in 2004. However, even without that year the trend is positive. The trends in the two regions are compatible with each other: the difference is not statistically significant. We therefore assume that the difference in trends is mainly due to natural variability. This is supported by the observation that the maxima in these two regions are uncorrelated. This corroborates our choice of the larger region as event definition. Averaging the two time series gives the best estimate for the observed trend in the region affected by storm Desmond. This gives an increase in probability of a factor 1.49–18.53 (95% confidence interval, best estimate 4.45, p = 0.008). The return period in the current climate is about 5 years (2.8 to 14 years confidence interval). Note that the assumptions made in the fit of equation (1) imply that the risk ratio is independent of the return period.
The Eskdalemuir series (figure 3) shows a strong increase in daily mean precipitation, in agreement with the Southern Scotland series (which likely includes this station). Again, the trend is already positive over the period. The 75.7 mm observed in one day has a return period of 2.6 to 13 years in the current climate, and the more relevant two-day sum of 139.9 mm a return period of 15 to 260 years (best estimate 51 years). At this location the heavy rain associated with storm Desmond was a fairly rare event even in the current climate. In the early 20th century, this fit indicates that it would have been even more rare, with a best estimate of the return period of hundreds of years and a lower bound of 89 years.
Figure 5. As figure 2 but now for the two HadGEM3-A 15 member ensemble experiments 1960–2015. (left) Experiment based on all forcings, (right) experiment based on natural forcing only, see text for details. The observed Desmond value is taken from ERA-Interim (25.15 mm, shifted to account for model bias).
Download figure:
Standard image High-resolution image4.2. Global climate models
We applied the same method on climate model data to decrease the statistical uncertainty at the expense of an increased systematic uncertainty. We used an experiment consisting of 16 ensemble members covering 1861–2016 of the EC-Earth 2.3 model (Hazeleger et al 2010) using the CMIP5 protocol (Taylor et al 2012). This model is very similar to the ECMWF seasonal forecasting model. The resolution is T159, about 150 km, which is too low to represent the mountains, where the highest precipitation in figure 1 is seen. We therefore only use the large area, 54–57°N, 6°W–2°W. Precipitation in this area shows a climatology comparable to ERA-Interim (which is made with a very similar model; Dee et al 2011). Extreme winter precipitation is concentrated in October–February, as in the observations. Furthermore, we use data from the UK Met Office HadGEM3-A model (Christidis et al 2013). The HadGEM3-A experiments were atmosphere-only but had much higher resolution (N216, about 60 km) and were integrated from 1960–2015 (15 ensemble members). There is a 'all forcing' experiment with sea surface temperatures (SSTs) and radiative forcing as observed and a 'natural' experiment in which the effects of climate change on SSTs and radiative forcing have been removed. For these experiments, the size and pattern of the climate change effects were taken from the CMIP5 ensemble.
Figure 4 shows the return periods of maximum daily winter precipitation in this area based on the EC-Earth simulations with a GEV fit under the same assumptions as for the observations. The figure shows an increase in the return period (a shift to the left in figure 4) for an extreme event of magnitude 25.15 mm day−1 as calculated from ERA-Interim (green horizontal line) due to the external forcings of a factor of 1.13−1.55 (95% CI, best estimate 1.29). This revised result is almost completely overlapping with the original estimates from EC-Earth (van Oldenborgh et al 2015) despite the adjusted return period.
The increase in return period can be translated to a shift in intensity (upwards shift) of such an event (Otto et al 2012). For heavy precipitation in Northern England in the EC-Earth model this is about 4%. The full CMIP5 ensemble for annual maxima (Sillmann et al 2013), a much softer extreme, gives a range of 3%–8% (interquartile range) using the methods of (van Oldenborgh et al 2013b) (not shown). We do not use this CMIP5 range in our synthesis as this ensemble includes many models with a resolution that is too low to resolve events like storm Desmond.
The increase in probability of these kinds of events in EC-Earth is in line with the observational one, although we expect a difference due to the different framing of the attribution question within the different methodologies. The observational analysis considers the change due to the observed trend, independent of the cause of this trend, while the coupled model shows the change due to the external anthropogenic and natural forcing prescribed in the model. The differences are mainly in the response to the aerosol and greenhouse gas forcings of the climate model used, which may differ somewhat from the real world. Very low frequency natural variability could also cause the results to diverge as this is averaged out more in the model ensemble than the single reality of the observations.
Another feature that will cause differences between the models and observations is the reduced orography in the EC-Earth model due to its relatively coarse resolution. This will lower variability somewhat as orography amplifies the effect of circulation (Burt and Howden 2013) and hence lead to an underestimation of the uncertainty. For the large area chosen, we expect the effect to be small for this winter situation. Higher-resolution models are needed to ascertain this. It should be noted that we also do not know the orographic situation of the stations that form the Northwest England and Southern Scotland time series and whether these are a good representation of the area average.
The analysis is repeated for the HadGEM3-A experiments. Combining the change of return periods in the two experiments for 1960–2015, 15 ensemble members each, the increase in the return period of an event like storm Desmond in 2015 is between 0.98 and 1.51 (95% C.I. best estimate 1.24) due to anthropogenic climate change (figure 5). The best estimate result is very close to that found for the EC-Earth experiment, though for HadGEM3-A the uncertainty range includes 1.00 (i.e. no change). This robust result increases our confidence in the result as two independent models and two experimental setups were used.
Figure 6. The maximum of winter (DJF) daily precipitation averaged over the Northern England/Southern Scotland, 54–57°N, 6°W–2°W. Red indicates the probability of daily mean precipitation under observed climate conditions, blue in the counterfactual simulations.
Download figure:
Standard image High-resolution image4.3. Large ensemble regional climate model simulations
The fact that the northern part of England is a mountainous region led to very heavy precipitation observed at some stations and almost none in neighbouring stations. Therefore, capturing the nature of the precipitation event requires relatively high resolution climate models that include local orography. Furthermore, using a very large ensemble it is not necessary to fit an extreme value distribution to analyse the rare events, hence no assumptions about the shape of the tails of the distribution are made, nor is the change in softer extremes related to the change in larger extremes.
Using the distributed computing framework weather@home, large ensembles of regional climate models at 50 km resolution over Europe are available for the last decade. Corresponding to these simulations of possible weather in Europe under current climate conditions ('all forcings'), ensembles of counterfactual simulations of possible weather in a world as it might have been without anthropogenic climate drivers ('natural') are run. As weather@home is an atmosphere-only modelling framework, observed SSTs are necessary to drive the model. SST for the 'natural' simulations is obtained by subtracting various estimates of the difference between preindustrial and present-day conditions from CMIP5 (Schaller et al 2014).
Figure 6 shows the return period of the winter maximum precipitation averaged over the area (54–57°N, 6°W–2°W) in the combined ensemble simulations of weather@home. The results are remarkably similar to those from the coupled model, in spite of slightly different definitions of seasons.
Previous studies using the same model in a very similar region have shown, however, that the weather@home model in the region is biased towards low precipitation values (Schaller et al 2016). This is not unexpected given that for heavy precipitation in mountainous, or at least hilly, terrain a resolution under 10 km would be needed to simulate the mechanisms leading to the heaviest rainfall. As before, we use the return period calculated from observations rather than the magnitude of the observed event. This leads to a more realistic estimate of changes in the likelihood of the occurrence of an event like the one observed on 5 December 2015, which is on the order of a one in five year event. In the weather@home ensemble simulations, the return period of a one in five year event in the current climate would have been an approximately one in ten (6−18) year event in a 'world that might have been' without anthropogenic climate change, increasing the likelihood of such an event to occur by a factor of 1.25 to 3.48.
Again, the question addressed with the atmosphere-only large ensemble method is slightly different from the other two methods. Here we ask how much the probability has changed given the influence of prescribed anthropogenic forcings and the observed SST patterns. Figure 6 shows the data for December–February (DJF) 2015/2016 while the other methods investigated a longer season (October–February). The best estimate in this case provides a higher increase in the likelihood of such an event occurring compared to the overall best estimate of 59% (calculated as the geometric mean over all methods considered) and compared to the real-time estimate of 40%. For the real-time analysis, an experiment using 2014/2015 data was used, suggesting that the SSTs observed at the time played indeed an important role. The differences are however not statistically significant. The other difference is that in these experiments the natural forcings are kept constant between the factual and counterfactual climates, whereas in the observations and coupled experiments they vary over time. However, these forcings have a small influence, as argued in the introduction, so that the results should be comparable between the different methods.
Figure 7. (a) Extreme value fit of the December precipitation sum in Northern England/Southern Scotland. The lines indicate a fit to a normal distribution, assuming the distribution scales with the smoothed observed global mean temperature. Red lines indicate the climate of 2015, blue lines the climate of 1920. The stars denotes the observed block maxima, shifted up with the fitted trend to 2015 (red) or down to 1920 (blue). The green line denotes the value observed in December 2015. (b) As for (a) but for the strength of El Niño. (c) As for (a) but for EC-Earth simulations (d) as for (b) but for EC-Earth simulations.
Download figure:
Standard image High-resolution image5. Monthly context
5.1. Observations
In addition to the daily data, we also analyse the monthly precipitation here. There is a very significant positive trend in average October–February in the Northern England/Southern Scotland region (UK Met Office NEE, NWE and SS time series) precipitation of about 20% up to 2016. This is much more than the increase from the Clausius–Clapeyron relation that describes the maximum amount of water vapour in the atmosphere. For a fixed relative humidity this gives an increase of about 6%–7% per degree warming in GMST. The larger increase in the observations is due to an increase in westerly circulation types (van Haren et al 2013b).
This trend also increases the probability of extreme monthly total rainfall, see figure 7(a). Here we fit a simple statistical model to the observed data: scaling the precipitation with the smoothed global mean temperature and describing the upper tail with a normal distribution, which fits quite well. This gives a return period in the current climate of about 128 years, with a 95% uncertainty range that starts at 18 years. In this model, the probability of observing such a precipitation extreme would have been extremely small in the climate of the beginning of the time series.
5.1.1. Connection with El Niño
Statistically, the Northern England precipitation has a weak connection with El Niño in December. The signal disappears in January and February, and reappears in March–April (van Oldenborgh 2000). In the coupled climate model EC-Earth, these correlations are absent (r = 0.01), so again our confidence that this is a physical signal and not a coincidence is very low.
Assuming the signal is real, the probability of an extreme winter month like the one observed increases somewhat due to the very strong El Niño of December 2015, see figures 7(b) and (d). However, the change in probability would be much less than the change associated with global warming (best estimate for ratios of change: 1200 for global warming, 150 for El Niño).
5.2. Global climate models
In the EC-Earth experiment, there is no December with precipitation exceeding the observed values of December 2015 (215 mm/month in ERA-Interim for the large area 6°W–2°W, 54–57°N). The estimated return period is many thousands of years (figure 7(d)). If we compare 2015 to the beginning of the time series this return period has increased by a factor between 1.06 and 8.51 (95% C.I. best estimate 2.24).
In the HadGEM3-A experiments, there is no significant trend towards increasing extreme monthly precipitation. The modeled ratio of return periods for the beginning of the time series and 2015 is 0.93−6.43 (95% C.I. best estimate 2.80) (not shown).
5.3. Large ensemble regional climate model simulations
A similar analysis in the weather@home simulations also indicate a difference between the factual and counterfactual simulations in Northern England/Southern Scotland precipitation for monthly data (not shown). Comparing 2015 to climatology simulations reveals greater precipitation in the 2015 simulations than in the climatology, showing that the particular SST patterns in 2015 favoured higher precipitation across the UK.
Using the example of a 1 in 100 (81–147) year event in Northern England from the Actual ensemble, the event becomes a 1 in 158 (123–220) year event in the counterfactual ensemble, and a 1 in 183 (153–230) year event in the Climatology ensemble. This corresponds roughly to a 50% increase in likelihood due to anthropogenic climate change and 75% increase in likelihood due to the SST patterns in 2015 relative to 1985–2013.
This indicates that the particular SSTs during 2015, such as the North Atlantic SSTs or the El Niño SST pattern, increased the likelihood of a wet December when looking at the month as a whole, and this effect is equal to or greater than the anthropogenic signal.
6. Discussion
6.1. Storm Desmond
When looking at the results of the real-time analysis, there was remarkable agreement between all three methods used to investigate the role of anthropogenic climate change in the type of heavy precipitation events associated with storm Desmond that passed over the northern part of the UK from 4–6 December 2015. It was found that climate change clearly increased the likelihood of large precipitation events in all three analyses. The likelihood of a 1 in a 100 year event of daily autumn/winter precipitation averaged over the land area of 54–57°N and 6°W–2°W increased by a factor of between 1.3–2.8 (95% confidence interval) based on the past trends in the observations, 1.1–1.8 in the coupled climate model EC-Earth and 1.05–1.4 in the large ensemble of regional climate model simulations (see table 1). The fits to the observations and the coupled model are dominated by the more frequent events and the assumption that the two distributions scale with the global mean temperature propagates that information to the upper tail. This assumption is not made in the large ensemble.
Revisiting this analysis here with a revised return period as well as updated data sets, the agreement is not as striking but the results are still very compatible with each other and with the real-time estimates. Looking at a one in five year event instead, we conclude that such events have increased by a factor of between 1.49–18.53 in observations, 1.13–1.55 in the EC Earth analysis, 0.98–1.51 in HadGEM3-A analysis and 1.25–3.48 in the large ensemble of weather@home. As in the real-time analysis, all methods show a statistically significant increase in risk in all but the HadGEM3-A analysis (which was not used in the real-time analysis), despite the fact that a much less rare event was analysed. The overall assessment of the change in likelihood due to anthropogenic climate change is therefore very similar to that of the real-time analysis. Excluding again the observations for the overall assessment but including this time the HadGEM3-A results leads to a best estimate of a 59% increase compared to a 40% increase in the real-time analysis. The increase in this estimate stems mainly from the much larger increase in likelihood in the 2015/2016 simulations in the large ensemble compared to the 2014/2015 estimates.
The comparably small increase in the likelihood of extreme rain observed as the return period increases, in the real-time analysis as well as now, shows that the scaling assumption in the GEV fitting method is not unreasonable in this case. Given the fact that all three applied methodologies frame the attribution question in a different way (e.g. Otto et al 2015, Uhe et al 2016) and that the station data includes the effects of local orography (although not necessarily in a way representative for that large area) that the climate models cannot capture, the quantification of the increase agrees well. This corroborates the assumption that this increase is indeed mainly due to anthropogenic climate forcings made in the observational analysis, and that the influence of other factors such as SST differences resulting from natural variability is small.
This analysis looks at the combined effect of a thermodynamically driven increase in precipitation and potential changes in the atmospheric circulation and thus gives an estimate of the overall change of the likelihood of occurrence of this type of event. The increase is compatible with a purely thermodynamic increase from Clausius–Clapeyron scaling caused by roughly a half degree warming of the upstream North Atlantic. The analysis is limited in that it only considers trends in precipitation and does not take into account other factors that influenced the flooding in Northern England, such as flood defences and increased exposure due to development in flood-prone areas (e.g. Crichton 2005).
6.2. Monthly context
The situation for precipitation is not entirely consistent between the methodologies in terms of the quantitative increase when looking at the monthly precipitation. The observational analysis gives a significant increase of orders of magnitude in the likelihood of a very wet December occurring over the past 140 years. For the EC-Earth simulations, we observe roughly a doubling in likelihood of the event. The weather@home simulations show an increase in the likelihood of a wet December in Northern England of approximately 50%. The statistical analysis of Northern England precipitation in December has a weak connection with the El Niño signal, but in the coupled model this connection is absent. For the weather@home modelling, we can note that the precipitation is lower in the Climatology simulations, indicating that the particular SSTs during 2015, such as the North Atlantic SSTs or the El Niño SST pattern, increased the likelihood of a wet December compared to an average month. For Northern England, this effect was equal to or greater than the anthropogenic signal; however, SST patterns similar to those of 2015 may also change in frequency with climate change. This modelling does not give an indication of whether there has been such a change in the likelihood of these types of SST.
7. Conclusion
During the days immediately after an extreme weather event with large impacts, like the floods in the UK following heavy rains around 5 December 2015, the question arises what role climate change has played. Using real-time observations and weather analyses, historical data, reanalyses and climate model output, we can now give a first scientific assessment of the effect of climate change in a relatively short time. The analysis here shows that the real-time estimates are robust for this kind of extreme weather event. For the analysis of the heavy precipitation event in Northern England and Southern Scotland caused by the storm Desmond we used three independent methods: a statistical analysis of observed trends, coupled climate model simulations and a large ensemble of regional climate model simulations. In this case, a lack of observations of the event precluded us from establishing the return period of the event with accuracy, but statements can be made about relative return periods under various scenarios. Based on the available data at the time, it appeared to be very roughly a one in one hundred year event when averaged over a large region, but with an uncertainty range from about 20 years to many hundreds of years. Locally return periods may be very different from this. With more observations becoming available a month and more after the event the return period was revised to be in the order of 5 years.
The increase in likelihood of the event does not depend strongly on the return period and was found to be in good agreement between the three methods.
For the event defined over the large region in section 2.1 to enable comparison with models and with the revised return period, the best estimate is 59% increase in risk with a larger uncertainty range that includes 0 and has an upper bound of 250%. Taking also into account that Desmond was not a one-off storm but was followed by two others also leading to extreme precipitation and flooding, the whole month of December was analysed as well, resulting in an increase in risk that is higher than the daily precipitation but also with larger quantitative discrepancies between the methodologies.
The reanalysis of a real-time attribution event shows that it is possible to provide a robust first-guess quantification of the role of anthropogenic climate change in an extreme rainfall event in a temperate climate zone with high day-to-day and low interannual variability. This is in particular important as it highlights that real-time attribution can be done even when station data of the event is not available. Putting the one day event in the context of a whole wet month also shows that the attribution question with respect to daily extremes is very different to that of very wet months. These results highlight again the strong dependency of attribution results on the event definition and thus the importance of accessible observational data.